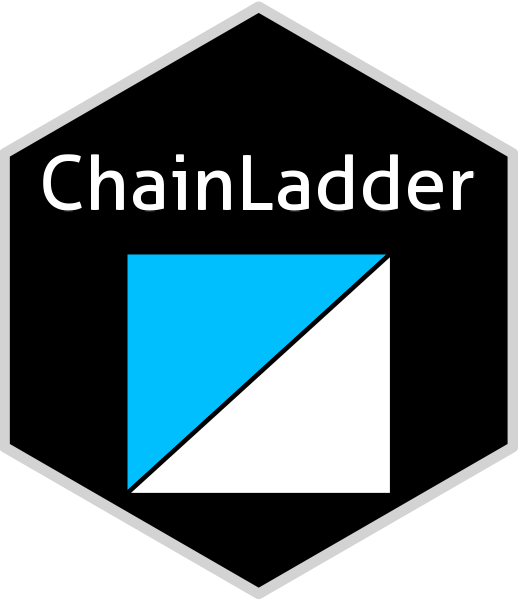
Estimate age-to-age factors
chainladder.Rd
Basic chain-ladder function to estimate age-to-age factors for a given cumulative run-off triangle. This function is used by Mack- and MunichChainLadder.
Arguments
- Triangle
cumulative claims triangle. A (mxn)-matrix \(C_{ik}\) which is filled for \(k \leq n+1-i; i=1,\ldots,m; m\geq n \), see
qpaid
for how to use (mxn)-development triangles with m<n, say higher development period frequency (e.g quarterly) than origin period frequency (e.g annual).- weights
weights. Default: 1, which sets the weights for all triangle entries to 1. Otherwise specify weights as a matrix of the same dimension as
Triangle
with all weight entries in [0; 1], where entry \(w_{i,k}\) corresponds to the point \(C_{i,k+1}/C_{i,k}\). Hence, any entry set to 0 orNA
eliminates that age-to-age factor from inclusion in the model. See also 'Details'.- delta
'weighting' parameters. Default: 1; delta=1 gives the historical chain-ladder age-to-age factors, delta=2 gives the straight average of the observed individual development factors and delta=0 is the result of an ordinary regression of \(C_{i,k+1}\) against \(C_{i,k}\) with intercept 0, see Barnett & Zehnwirth (2000).
Please note that
MackChainLadder
uses the argumentalpha
, withalpha = 2 - delta
, following the original paper Mack (1999)
Details
The key idea is to see the chain-ladder algorithm as a special form of a weighted linear regression through the origin, applied to each development period.
Suppose y
is the vector of cumulative claims at development period
i+1
, and x
at development period i
, weights
are
weighting factors and F
the individual age-to-age factors F=y/x
. Then
we get the various age-to-age factors:
Basic (unweighted) linear regression through the origin:
lm(y~x + 0)
Basic weighted linear regression through the origin:
lm(y~x + 0, weights=weights)
Volume weighted chain-ladder age-to-age factors:
lm(y~x + 0, weights=1/x)
Simple average of age-to-age factors:
lm(y~x + 0, weights=1/x^2)
Barnett & Zehnwirth (2000) use delta = 0, 1, 2 to distinguish between the above
three different regression approaches: lm(y~x + 0, weights=weights/x^delta)
.
Thomas Mack uses the notation alpha = 2 - delta
to achieve the same result:
sum(weights*x^alpha*F)/sum(weights*x^alpha) # Mack (1999) notation
Value
chainladder returns a list with the following elements:
- Models
linear regression models for each development period
- Triangle
input triangle of cumulative claims
- weights
weights used
- delta
deltas used
References
Thomas Mack. The standard error of chain ladder reserve estimates: Recursive calculation and inclusion of a tail factor. Astin Bulletin. Vol. 29. No 2. 1999. pp.361:366
G. Barnett and B. Zehnwirth. Best Estimates for Reserves. Proceedings of the CAS. Volume LXXXVII. Number 167. November 2000.
See also
See also
ata
,
predict.ChainLadder
MackChainLadder
,
Examples
## Concept of different chain-ladder age-to-age factors.
## Compare Mack's and Barnett & Zehnwirth's papers.
x <- RAA[1:9,1]
y <- RAA[1:9,2]
F <- y/x
## wtd. average chain-ladder age-to-age factors
alpha <- 1 ## Mack notation
delta <- 2 - alpha ## Barnett & Zehnwirth notation
sum(x^alpha*F)/sum(x^alpha)
#> [1] 2.999359
lm(y~x + 0 ,weights=1/x^delta)
#>
#> Call:
#> lm(formula = y ~ x + 0, weights = 1/x^delta)
#>
#> Coefficients:
#> x
#> 2.999
#>
summary(chainladder(RAA, delta=delta)$Models[[1]])$coef
#> Estimate Std. Error t value Pr(>|t|)
#> x 2.999359 1.130203 2.653822 0.02908283
## straight average age-to-age factors
alpha <- 0
delta <- 2 - alpha
sum(x^alpha*F)/sum(x^alpha)
#> [1] 8.206099
lm(y~x + 0, weights=1/x^(2-alpha))
#>
#> Call:
#> lm(formula = y ~ x + 0, weights = 1/x^(2 - alpha))
#>
#> Coefficients:
#> x
#> 8.206
#>
summary(chainladder(RAA, delta=delta)$Models[[1]])$coef
#> Estimate Std. Error t value Pr(>|t|)
#> x 8.206099 4.113487 1.994925 0.08115167
## ordinary regression age-to-age factors
alpha=2
delta <- 2-alpha
sum(x^alpha*F)/sum(x^alpha)
#> [1] 2.217241
lm(y~x + 0, weights=1/x^delta)
#>
#> Call:
#> lm(formula = y ~ x + 0, weights = 1/x^delta)
#>
#> Coefficients:
#> x
#> 2.217
#>
summary(chainladder(RAA, delta=delta)$Models[[1]])$coef
#> Estimate Std. Error t value Pr(>|t|)
#> x 2.217241 0.4112176 5.391893 0.0006522995
## Compare different models
CL0 <- chainladder(RAA)
## age-to-age factors
sapply(CL0$Models, function(x) summary(x)$coef["x","Estimate"])
#> [1] 2.999359 1.623523 1.270888 1.171675 1.113385 1.041935 1.033264 1.016936
#> [9] 1.009217
## f.se
sapply(CL0$Models, function(x) summary(x)$coef["x","Std. Error"])
#> [1] 1.130203277 0.135836119 0.090498216 0.025389927 0.035376679 0.022577813
#> [7] 0.004881918 0.015055851 NaN
## sigma
sapply(CL0$Models, function(x) summary(x)$sigma)
#> [1] 166.983470 33.294538 26.295300 7.824960 10.928818 6.389042 1.159062
#> [8] 2.807704 NaN
predict(CL0)
#> dev
#> origin 1 2 3 4 5 6 7 8
#> 1981 5012 8269.000 10907.000 11805.00 13539.00 16181.00 18009.00 18608.00
#> 1982 106 4285.000 5396.000 10666.00 13782.00 15599.00 15496.00 16169.00
#> 1983 3410 8992.000 13873.000 16141.00 18735.00 22214.00 22863.00 23466.00
#> 1984 5655 11555.000 15766.000 21266.00 23425.00 26083.00 27067.00 27967.34
#> 1985 1092 9565.000 15836.000 22169.00 25955.00 26180.00 27277.85 28185.21
#> 1986 1513 6445.000 11702.000 12935.00 15852.00 17649.38 18389.50 19001.20
#> 1987 557 4020.000 10946.000 12314.00 14428.00 16063.92 16737.55 17294.30
#> 1988 1351 6947.000 13112.000 16663.88 19524.65 21738.45 22650.05 23403.47
#> 1989 3133 5395.000 8758.905 11131.59 13042.60 14521.43 15130.38 15633.68
#> 1990 2063 6187.677 10045.834 12767.13 14958.92 16655.04 17353.46 17930.70
#> dev
#> origin 9 10
#> 1981 18662.00 18834.00
#> 1982 16704.00 16857.95
#> 1983 23863.43 24083.37
#> 1984 28441.01 28703.14
#> 1985 28662.57 28926.74
#> 1986 19323.01 19501.10
#> 1987 17587.21 17749.30
#> 1988 23799.84 24019.19
#> 1989 15898.45 16044.98
#> 1990 18234.38 18402.44
CL1 <- chainladder(RAA, delta=1)
## age-to-age factors
sapply(CL1$Models, function(x) summary(x)$coef["x","Estimate"])
#> [1] 2.999359 1.623523 1.270888 1.171675 1.113385 1.041935 1.033264 1.016936
#> [9] 1.009217
## f.se
sapply(CL1$Models, function(x) summary(x)$coef["x","Std. Error"])
#> [1] 1.130203277 0.135836119 0.090498216 0.025389927 0.035376679 0.022577813
#> [7] 0.004881918 0.015055851 NaN
## sigma
sapply(CL1$Models, function(x) summary(x)$sigma)
#> [1] 166.983470 33.294538 26.295300 7.824960 10.928818 6.389042 1.159062
#> [8] 2.807704 NaN
predict(CL1)
#> dev
#> origin 1 2 3 4 5 6 7 8
#> 1981 5012 8269.000 10907.000 11805.00 13539.00 16181.00 18009.00 18608.00
#> 1982 106 4285.000 5396.000 10666.00 13782.00 15599.00 15496.00 16169.00
#> 1983 3410 8992.000 13873.000 16141.00 18735.00 22214.00 22863.00 23466.00
#> 1984 5655 11555.000 15766.000 21266.00 23425.00 26083.00 27067.00 27967.34
#> 1985 1092 9565.000 15836.000 22169.00 25955.00 26180.00 27277.85 28185.21
#> 1986 1513 6445.000 11702.000 12935.00 15852.00 17649.38 18389.50 19001.20
#> 1987 557 4020.000 10946.000 12314.00 14428.00 16063.92 16737.55 17294.30
#> 1988 1351 6947.000 13112.000 16663.88 19524.65 21738.45 22650.05 23403.47
#> 1989 3133 5395.000 8758.905 11131.59 13042.60 14521.43 15130.38 15633.68
#> 1990 2063 6187.677 10045.834 12767.13 14958.92 16655.04 17353.46 17930.70
#> dev
#> origin 9 10
#> 1981 18662.00 18834.00
#> 1982 16704.00 16857.95
#> 1983 23863.43 24083.37
#> 1984 28441.01 28703.14
#> 1985 28662.57 28926.74
#> 1986 19323.01 19501.10
#> 1987 17587.21 17749.30
#> 1988 23799.84 24019.19
#> 1989 15898.45 16044.98
#> 1990 18234.38 18402.44
CL2 <- chainladder(RAA, delta=2)
## age-to-age factors
sapply(CL2$Models, function(x) summary(x)$coef["x","Estimate"])
#> [1] 8.206099 1.695894 1.314510 1.182926 1.126962 1.043328 1.034355 1.017995
#> [9] 1.009217
## f.se
sapply(CL2$Models, function(x) summary(x)$coef["x","Std. Error"])
#> [1] 4.113487235 0.167616428 0.119849168 0.027269226 0.033389333 0.025122915
#> [7] 0.004953969 0.015093015 NaN
## sigma
sapply(CL2$Models, function(x) summary(x)$sigma)
#> [1] 12.340461705 0.474090850 0.317091093 0.066795689 0.074660819
#> [6] 0.050245830 0.008580526 0.021344747 NaN
predict(CL2)
#> dev
#> origin 1 2 3 4 5 6 7 8
#> 1981 5012 8269.00 10907.000 11805.00 13539.00 16181.00 18009.00 18608.00
#> 1982 106 4285.00 5396.000 10666.00 13782.00 15599.00 15496.00 16169.00
#> 1983 3410 8992.00 13873.000 16141.00 18735.00 22214.00 22863.00 23466.00
#> 1984 5655 11555.00 15766.000 21266.00 23425.00 26083.00 27067.00 27996.90
#> 1985 1092 9565.00 15836.000 22169.00 25955.00 26180.00 27314.32 28252.71
#> 1986 1513 6445.00 11702.000 12935.00 15852.00 17864.61 18638.64 19278.97
#> 1987 557 4020.00 10946.000 12314.00 14566.55 16415.95 17127.21 17715.62
#> 1988 1351 6947.00 13112.000 17235.86 20388.74 22977.34 23972.89 24796.49
#> 1989 3133 5395.00 9149.351 12026.92 14226.95 16033.23 16727.91 17302.61
#> 1990 2063 16929.18 28710.107 37739.73 44643.30 50311.31 52491.18 54294.53
#> dev
#> origin 9 10
#> 1981 18662.00 18834.00
#> 1982 16704.00 16857.95
#> 1983 23888.27 24108.44
#> 1984 28500.70 28763.38
#> 1985 28761.12 29026.20
#> 1986 19625.90 19806.78
#> 1987 18034.42 18200.63
#> 1988 25242.70 25475.36
#> 1989 17613.97 17776.31
#> 1990 55271.56 55780.98
## Set 'weights' parameter to use only the last 5 diagonals,
## i.e. the last 5 calendar years
calPeriods <- (row(RAA) + col(RAA) - 1)
(weights <- ifelse(calPeriods <= 5, 0, ifelse(calPeriods > 10, NA, 1)))
#> [,1] [,2] [,3] [,4] [,5] [,6] [,7] [,8] [,9] [,10]
#> [1,] 0 0 0 0 0 1 1 1 1 1
#> [2,] 0 0 0 0 1 1 1 1 1 NA
#> [3,] 0 0 0 1 1 1 1 1 NA NA
#> [4,] 0 0 1 1 1 1 1 NA NA NA
#> [5,] 0 1 1 1 1 1 NA NA NA NA
#> [6,] 1 1 1 1 1 NA NA NA NA NA
#> [7,] 1 1 1 1 NA NA NA NA NA NA
#> [8,] 1 1 1 NA NA NA NA NA NA NA
#> [9,] 1 1 NA NA NA NA NA NA NA NA
#> [10,] 1 NA NA NA NA NA NA NA NA NA
CL3 <- chainladder(RAA, weights=weights)
summary(CL3$Models[[1]])$coef
#> Estimate Std. Error t value Pr(>|t|)
#> x 3.47986 1.060538 3.281222 0.04638316
predict(CL3)
#> dev
#> origin 1 2 3 4 5 6 7 8
#> 1981 5012 8269.00 10907.00 11805.00 13539.00 16181.00 18009.00 18608.00
#> 1982 106 4285.00 5396.00 10666.00 13782.00 15599.00 15496.00 16169.00
#> 1983 3410 8992.00 13873.00 16141.00 18735.00 22214.00 22863.00 23466.00
#> 1984 5655 11555.00 15766.00 21266.00 23425.00 26083.00 27067.00 27967.34
#> 1985 1092 9565.00 15836.00 22169.00 25955.00 26180.00 27277.85 28185.21
#> 1986 1513 6445.00 11702.00 12935.00 15852.00 17435.13 18166.26 18770.54
#> 1987 557 4020.00 10946.00 12314.00 14259.49 15683.57 16341.26 16884.83
#> 1988 1351 6947.00 13112.00 16600.64 19223.37 21143.20 22029.83 22762.62
#> 1989 3133 5395.00 10318.43 13063.80 15127.75 16638.55 17336.28 17912.95
#> 1990 2063 7178.95 13730.40 17383.58 20130.01 22140.38 23068.83 23836.18
#> dev
#> origin 9 10
#> 1981 18662.00 18834.00
#> 1982 16704.00 16857.95
#> 1983 23863.43 24083.37
#> 1984 28441.01 28703.14
#> 1985 28662.57 28926.74
#> 1986 19088.45 19264.38
#> 1987 17170.80 17329.05
#> 1988 23148.14 23361.48
#> 1989 18216.33 18384.22
#> 1990 24239.88 24463.29